“Prediction Error‑Driven Memory Consolidation for Continual Learning: On the Case of Adaptive Greenhouse Models” is finally out and open access in Springer KI (German journal on Artificial Intelligence), special issue on developmental robotics! Check it here: https://link.springer.com/article/10.1007/s13218-020-00700-8
Springer journal on Künstliche Intelligenz
Happy that my paper with L.Miranda and U. Schmidt on “Prediction error-driven memory consolidation for continual learning. On the case of adaptive greenhouse models” has been accepted for publication in the Springer German journal of Artificial Intelligence (Springer KI)! Pre-print available here.
Acta Horticulturae
My work with Luis Miranda (HNEE Eberswalde) on adaptive architectures for portability of greenhouse models has been finally published in Acta Horticulturae!
DOI 10.17660/ActaHortic.2020.1296.4 . Pre-print available here: https://arxiv.org/pdf/1908.01643.pdf

IEEE ICDL Epirob 2020
My talk on “Tracking Emotions: Intrinsic Motivation Grounded on Multi-Level Prediction Error Dynamics”, presented at IEEE International Conference on Development and Learning and Epigenetic Robotics is online!
Accompanying paper: https://arxiv.org/abs/2007.14632
Accompanying code: https://github.com/guidoschillaci/prediction_error_dynamics
iCub multimodal dataset paper accepted for publication at ACM ICMI 2020
Just notified that our paper describing the iCub multisensor datasets for robot and computer vision applications (work of Murat Kirtay (HU-Berlin), of colleagues at the BioRobotics Institute (SSSA) and myself) has been accepted for publication at the 22nd ACM International Conference on Multimodal Interaction (ICMI2020).
Pre-print available here.
Tracking emotions: intrinsic motivation grounded on multi-level prediction error dynamics
Happy that my paper on “Tracking emotions: intrinsic motivation grounded on multi-level prediction error dynamics”, co-authored with Alejandra Ciria (UNAM, MX) and Bruno Lara (UAEM, MX), has been accepted for presentation at IEEE ICDL-Epirob 2020!
In this work, we propose a learning architecture that generates exploratory behaviours towards self-generated goals in a simulated robot, and that regulates goal selection and the balance between exploitation and exploration through a multi-level monitoring of prediction error dynamics.
The system is made of: 1) a convolutional autoencoder for unsupervised learning of low-dimensional features from visual inputs; 2) a self-organising map for online learning of visual goals; two deep neural networks, trained in an online fashion, encoding controller and predictor of the system. Memory replay is employed to face catastrophic forgetting issues.
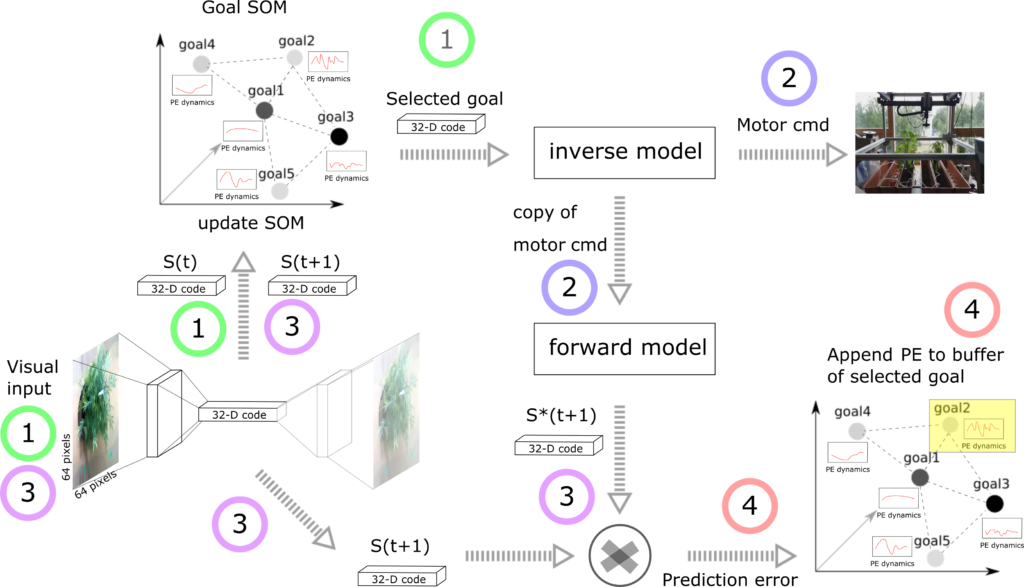
A multi-level monitoring mechanism keeps track of two errors: (1) a high-level, general error of the system, i.e. MSE of the forward model calculated on a test dataset; (2) low-level goal errors, i.e. the prediction errors estimated when trying to reach each specific goal.
The system maintains a buffer of high-level MSE observed during a specific time window. After every update of the MSE buffer, a linear regression is calculated on the stored values over time, whose slope indicates the trend of the general error of the system.
This trend modulates computational resources (size of goal error buffers) and exploration noise: when overall performances improve, the necessity of tracking the goal error dynamics is reduced. On the contrary, the system widens the time window on which goal errors are monitored.
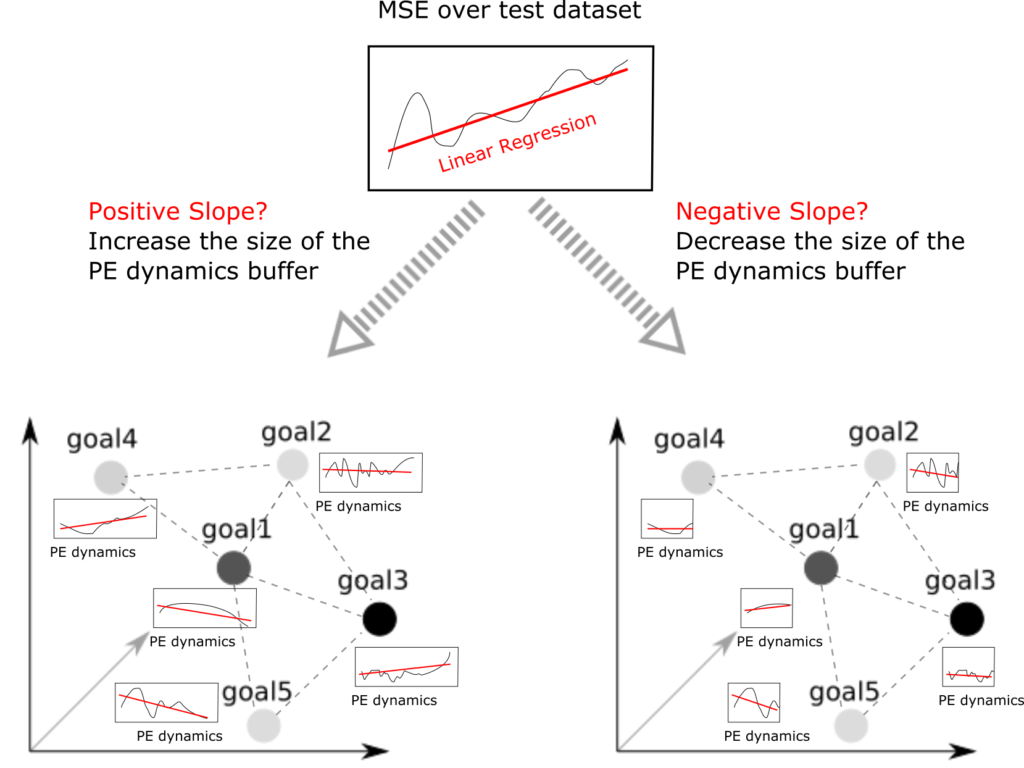
We discuss the tight relationship that PE dynamics may have with the emotional valence of action. PE dynamics may be fundamental cause of emotional valence of action: positive valence linked to an active reduction of PE and a negative valence to a continuous increase of PE.
Read the full paper here: https://arxiv.org/abs/2007.14632 (pre-print)!
Prediction-error driven memory consolidation for continual learning and adaptive greenhouse models
Check my AI Transfer work submitted to Springer KI (German Journal on Artificial Intelligence, special issue on Developmental Robotics) on “Prediction error-driven memory consolidation for continual learning”, applied on data from innovative greenhouses: https://arxiv.org/abs/2006.12616.
Episodic memory replay and prediction-error driven consolidation are used to tackle online learning in deep recurrent neural networks. Inspired by evidences from cognitive sciences and neuroscience, memories are retained depending on their congruency with prior knowledge.
This congruency is estimated in terms of prediction errors resulting from a generative model. In particular, our framework chooses which samples to maintain in the episodic memory based on their expected contribution to the learning progress.
Different retention strategies are compared. We analyse their impact on the variance of the samples stored in the memory and on the stability/plasticity of the model.
Co-authored with Luis Miranda and Uwe Schmidt, Humboldt-Universität zu Berlin.
SAGE Adaptive Behavior
Our article on “Intrinsic Motivation and Episodic Memories for Robot Exploration of High-Dimensional Sensory Spaces” is out in SAGE Adaptive Behaviour! Pre-print available here: https://arxiv.org/abs/2001.01982
Co-authored with Antonio Pico (HU-Berlin), Verena Hafner (HU-Berlin), Peter Hanappe (Sony CSL), David Colliaux (Sony CSL) and Timothee Wintz (Sony CSL)
iCub multisensor datasets
The iCub multisensor datasets for robot and computer vision applications produced by Murat Kirtay (SCIoI, Germany) and colleagues from Sant’Anna is out! ArXiv dataset report available here.
Frontiers in Neurorobotics
Our paper on prerequisites for an artificial Self has been published in Frontiers in Neurorobotics!